There’s no question that artificial intelligence (AI) and machine learning (ML) have changed the insurance landscape and will continue to do so. These technologies have provided new analytical approaches necessary to solve problems that weren’t possible through older traditional means. However, as with most new technologies, the conceptual discussion of its benefits is often idealistic, while real-world implementations sometimes fall short. While the vision of the benefits of AI/ML serves an essential purpose of suggesting what’s possible, realizing a return on AI/ML requires a comprehensive data strategy and well-implemented data-management infrastructure.
Data is the new currency
A stellar team of data scientists and ML engineers can’t be leveraged successfully if the foundation for their work doesn’t exist. Any application or attempt at AI and ML is only as good as the underlying data. Monica Rogati, independent data science and AI advisor and Equity Partner of venture capital firm Data Collective (DCVC) made the analogy to Maslow’s Hierarchy of Needs. The most basic and essential needs (such as food, water, and shelter) are at the bottom and lay the foundation for the “growth” needs (self-actualization), which are at the top of the pyramid. While Monica created this analogy back in 2017, it is still a relevant framework for ‘data as the new gold.’ Translating that framework to AI and data science, it’s necessary to collect, manage, cleanse, and label data before AI projects can be successfully pursued.
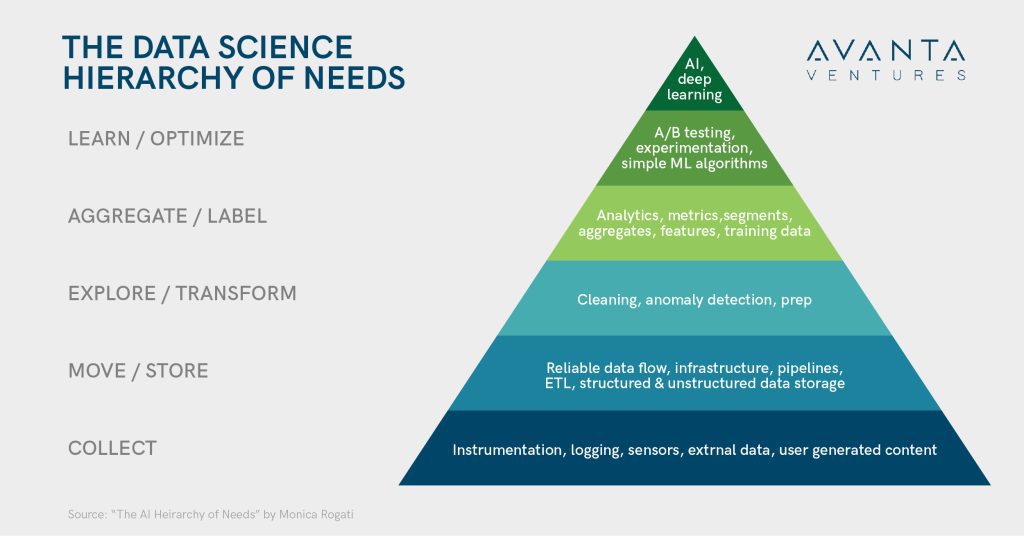
Building the foundation
At the very bottom of the pyramid is data collection. The greatest asset to any business, especially an insurance carrier, is the unique set of data that they’ve collected and maintained, especially claims loss data. The key is getting the most out of the data we have access to, knowing our data limitations, and having a path to supplement and augment it with external data. The questions to ask ourselves when trying to better understand our existing internal and external data are:
- What data are necessary to make critical business decisions, and do we have access to them?
- Are we capturing and logging all relevant data available in-house?
- What data are we missing in-house, and how can we best acquire it?
As we understand our data, we can identify what types of external and third-party data we need to supplement and add to our data to complete analyses. Leveraging third-party data isn’t a novel concept, but the breadth of the types of data that can be brought in, as well as the ability to process said data, has changed dramatically in the past few years.
Companies like Verisk and LexisNexis are veteran data and score suppliers to the industry and have partnered with insurers for years. Newer players have also emerged leveraging novel data sources, like Cape Analytics with its geospatial data, Motorq with its auto OEM data, DynaRisk with its data on customer cyber-risk profiles, Socialeads with its data predicting important life events, and Arity with its auto telematics and loss data.
In addition to companies that provide third-party structured data, there are also players whose mission is to help extract the full value of in-house unstructured data. Hyperscience automates manual document processing and converts unstructured documents, like PDFs, into structured data. Tractable extracts critical information, such as the amount of damage from images, for use in claims-processing automation. Both are examples of solutions that augment and improve in-house data. Both are also AI companies, so it’s necessary to have a data infrastructure that can adequately support and integrate their extracted data before partnering with them.
The concept of integrating in-house and external data has also evolved from how carriers initially worked with third-party data, thanks to newer, data-first carriers like Next Insurance. Many carriers often housed third-party data in disparate locations or kept it as unstructured data files that were accessed as needed. Due to the cumbersome efforts required to build custom integrations, they integrated third-party and in-house data only when necessary. This often involved creating a separate dashboard and importing what were believed to be relevant data points to conduct any analyses on the external data. However, it’s crucial to have the capability to easily integrate the data into a unified data-management platform so that it can be experimented with and explored fluidly in conjunction with in-house data.
Beyond third-party data: Data ecosystems
The other benefit of having a stable data foundation is the ability to develop and participate in data ecosystems. Data ecosystems are typically sector or problem-specific collaborations where organizations share data, most typically through federated analytics (decentralized analytics). While the concept hasn’t quite come over into insurance, industry data co-ops and open data ecosystems have been shown to help participants share insights and provide better offerings. Examples include Airbus’ Skywise and the Open Manufacturing Platform established by BMW and Microsoft. The biggest challenges around federated data ecosystems are data ownership, compliance, privacy, ethics, and trust issues. However, they are becoming more prevalent and prime examples of next-generation value creation by organizations collaborating and innovating together around data.
Creating the right infrastructure
Having a useful data infrastructure and understanding our data usage needs go hand-in-hand. Logging and collecting the correct data and identifying the right sources to augment your data are just part of the process. Ensuring that the data is stored and labeled correctly while flowing through the system reliably is essential. It enables us to explore and transform that data confidently.
Years of legacy systems and the introduction of newer platforms that don’t always speak to legacy systems can result in a complex mess of multiple discordant and disparate data sets linked through bespoke connections that are difficult to coordinate. Solutions like “data lakes” and “data warehouses” are great in concept but tend to be multiyear and multimillion-dollar undertakings, which often makes carriers and financial institutions lethargic.
Despite upfront costs, the benefits of a robust and flexible data management system are necessary, as mentioned before, to lay the foundation for analytics and AI in the future. A solid data infrastructure enables the organization to become data literate. It also allows the organization to incorporate the raw data and the resulting insights into one location. This allows for easier sharing of ideas and collaboration throughout the organization for a more holistic and data-driven approach to problem-solving. Breaking down data silos within an organization also allows for the ability to transition from solely a product-based view within the data to a product- and customer-centric understanding of the data across the entire organization.
Ensuring data quality
A good data infrastructure enables the organization to identify data gaps and solve for data quality issues. Data quality, especially in a regulated industry like ours, plays a key role in frontline business decisions. If we can’t trust the data. then as a responsible insurer, we cannot leverage that data, especially when it comes to underwriting decisions based on that data putting us at a pricing disadvantage. That’s why data governance, documentation, normalization, and validation are so important.
Something as simple as a lack of consistency in addresses can lead to poor data flow and integration by generating duplicate instances and confusion. Collecting partial or inconsistent records can cause issues with algorithms or uncertainty integrating external data with in-house data. Continuing with the varying address inputs as an example, let’s say the address “111 W 1st St” is also recorded as “111 West First Street.” Imagine creating an algorithm dependent upon leveraging claims data tied to those addresses to identify which locations are more or less risky. If you have enough of those inconsistencies, you can see how the data could potentially start to skew the algorithm and its results. A human might correlate those two addresses, but manual processes don’t scale. Normalizing the data for such a trivial variance is technically challenging and results in data scientists spending more of their expensive bandwidth cleansing data instead of deriving business insight from the data.
Importance of timeliness of data
Another important capability of a solid infrastructure is capturing large volumes of data and maintaining that as close to real-time as possible. The efficacy of some analyses is highly dependent on the ability to access current data to react to changes due to new circumstances. Look no further than the COVID-19 pandemic as an example.
Importance of context
Data may not lie, but it can mislead. That’s where context comes in. Building context into AI and ML models remains a challenge. The nuances must be captured and documented in the underlying data so we’re able to identify biases and separate out correlation vs. causation.
Is the purchase of a carbon monoxide detector predictive of a responsible individual, or does that signal only hold meaning if the detector has been installed? Differentiating correlation from causation is important. One example of context being key to understanding the value of data is if we look at permits as a predictive indicator of the responsibility of a homeowner. In theory, the more permits a homeowner has pursued, the more risk-averse that homeowner is. However, permit requirements vary at a city level, so a blanket rule without context could result in incorrect pricing and underwriting. Lack of context and data bias has landed quite a few companies in the news, from inadvertent racial bias in an algorithm used by healthcare institutions and insurance carriers to accidental gender bias in Amazon’s hiring algorithm.
Context can also help prompt new questions and perspectives to identify shortcomings in data. However, incorporating context is difficult, especially as organizations move toward a “data lake” or a large data repository infrastructure. In addition to the challenges of overcoming complex legacy and bespoke data infrastructure, maintaining context without overcomplicating the data remains one of the biggest challenges to transforming an organization’s data infrastructure and flow.
What does this mean for the future?
While insurance companies have always been data-rich, the difference between traditional carriers and newer players like Kin is that the latter were built to be data-first and AI-first, which means prioritizing data quality, infrastructure, and data literacy. Many carriers try to plug in AI solutions under the strong influence of the AI and ML hype and attempt to keep up with AI-focused companies like Lemonade. They hope that’ll make up for and magically fix the shortcomings of their legacy data foundation and infrastructure. Unfortunately, despite what some AI and ML solutions claim, having a solid data foundation is necessary to get the most out of AI and ML tools. Tech giants like Google, Amazon, and Facebook dominated their industries by building data-enabled product and business model barriers to entry on top of robust, scalable, and highly performant data-management infrastructures.
So, what happens once we do have that foundation? Well, that’s where the journey truly starts. While a performant data management foundation enables the pursuit of AI and ML projects, those analytic tools still need to be applied thoughtfully. AI and ML are not an analytical panacea that solve all problems but are powerful tools when applied appropriately. It’s important to identify what types and which data-enabled analytic solutions best address the needs of your organization, whether that’s solution- and industry-specific AI and analytic solutions like FRISS or more generic, AI-enablement tools like DataRobot and SymetryML, which we’ll dive into in the future.
What are your thoughts? Let us know in the comments.