The world of artificial intelligence (AI) is currently experiencing a unique moment, thanks to the latest advances in generative AI. Not only does generative AI offer powerful tools for analyzing and generating new content, but it also democratizes AI, providing increased access for individuals and businesses. This brings new potential to how AI, especially generative AI, can transform industries and how people work, including in insurance. Advanced AI models can provide valuable insights, including analyzing large amounts of unstructured data and generating new content, leading to improved underwriting and pricing decisions based on better analytics, enhanced customer experiences and higher customer satisfaction; and streamlined business processes and improved workflow efficiency. However, fully capitalizing on the potential of generative AI requires further advancement due to the limitations of these technologies.
A quick introduction
Artificial intelligence, generative AI, and, most notably, ChatGPT have captured the general population’s imagination and high valuations from venture investors. Applications of these technologies can potentially transform how work is performed in many industries, including insurance.
Generative AI and ChatGPT are often used interchangeably, but they are different. ChatGPT is an application that is built on top of generative AI models. Generative AI is a subset of artificial intelligence consisting of numerous models that generate new content, such as text, images, videos, or sounds, based on patterns learned from existing data. This area of AI is rapidly evolving and has gained significant attention due to advancements in deep learning techniques that have enabled more sophisticated and effective generative models.
Most of the prevalent AI models fall into a category of models called discriminative:
- Discriminative AI classifies examples using attributes and metadata. It will identify patterns to distinguish the line between data categories. For example, a discriminative model can determine the probability of an animal being a cat or dog due to the appearance or lack of appearance of one or more identified attributes, such as pointy ears, which means that the image is one of a cat.
- Generative AI maps the distribution of attributes on examples. It will determine the combination of patterns and structures that define different categories. In the same cat versus dog example, a generative model will have used the examples labeled cats to map the typical attributes associated with a cat to create a new example of a cat.
Generative AI can identify patterns and connections within data inputs to gain a contextual understanding of an object or situation. As a result, generative AI models generally possess a more comprehensive understanding of the underlying data than discriminative models. On the other hand, discriminative models are specifically trained to address particular problems and, as a result, they may have a deeper and more accurate ability to tackle those specific tasks.
Limits of generative AI models
While generative AI has tremendous potential and while adoption has exploded, there are still numerous flaws and limits to the models as they exist today. Users should be wary of and understand these limits and what that means for potential implementations that leverage this technology, including:
- Generative AI models like ChatGPT and similar models are designed to mimic and create based on recognized patterns. They are not designed for accuracy, leading to content that seems real but may be false.
For instance, large language models (LLMs) such as ChatGPT are at their core predictive models that decide the next best word without considering the information’s accuracy. This can lead to a “hallucination effect” at times, where the output accuracy deviates further during extended conversations to provide a cohesive “story.” Since generative AI models are designed to mimic, they are also limited to mimicking the data they’ve learned from. When faced with new information not found within its database, it would either be unable to produce a result or could end up “hallucinating” a response.
- Generative AI models require massive datasets, usually at the scale of terabytes or petabytes.
The breadth and depth of the training data make generative AI capable of achieving its feats. However, due to the scale of the data, it is challenging to maintain the quality and accuracy of the underlying data, which could lead to misinformation and inherent flaws in the model. Models also perpetuate and amplify biases and prejudices present in the training data, which can be harder to root out given the scale of the data for generative AI models.
- The size of generative AI models requires heavy computing power to support it.
Today’s computing power required to build and run generative models makes it cost-prohibitive. Although workarounds have emerged, they are inherently built on the generalized model, meaning content leakages still happen.
- Explaining the outputs of generative AI models can be challenging, if not impossible.
Due to the scale of the underlying data and the nature of the generative model, it can be difficult to pinpoint the rationale behind a specific output. Lack of explainability will also challenge adoption as regulations start to be structured around the technology.
- Generative AI can be highly variable and difficult to control.
Generative AI models are inherently fluid and subject to variability. There is no guarantee that the same prompt will result in the same answer in subsequent attempts. Small nuances in inputs can result in amplified effects on the outputs. As a result, with the current capabilities of generative AI, most implementations require a human in the loop.
- Many models, including ChatGPT, leverage reinforcement learning and are hosted on the cloud.
Because most generative models undergo reinforcement learning, questions posed and data entered are stored and ultimately used to retrain the model in the future. The inputs are completely out of the user’s control. This could pose a risk for some enterprises if they don’t have their private instance of the model, especially when dealing with personally identifiable information. As a result, some use cases where data regulations are more stringent may be further out until on-premises solutions are more feasible and cost-effective.
Applications today and the potential of generative AI
Despite the limitations, if leveraged with care, there are still countless ways that generative AI can be used today. Companies are leveraging generative AI to create all sorts of media and text, including code. CB Insights has created a generative AI market map that splits the landscape into multiple categories and subcategories. Some categories include visual media generation, speech and audio generation, code generation, industry-specific companies, and cross-industry companies. The following is a portion of that map that focuses on text generation companies, providing a glimpse into the scale of the market and use cases of generative AI:
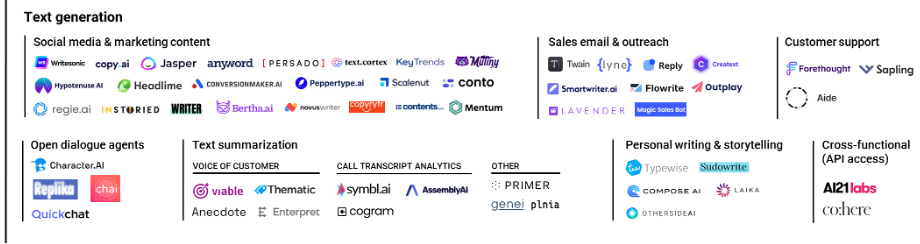
CB Insights’ market map of text generation startups leveraging generative AI
What’s especially exciting about generative AI and why it lends itself to many different use cases is not just the technology’s versatility but how generative AI democratizes AI. It has made AI more accessible to people who do not have a background in computer science or machine learning.
Although powerful, the models are heavily limited, as mentioned earlier. The potential of these models replacing actual humans and jobs is unlikely to occur any time soon. What can be expected is situations where “underwriters” are replaced with “underwriters leveraging AI.” Understanding how to leverage and work with AI and become the human in the “loop” will become more mainstream, especially since coding will not necessarily be a requisite to leverage AI.
For carriers, generative AI has potential applications throughout the insurance value chain. Below are just a few examples:
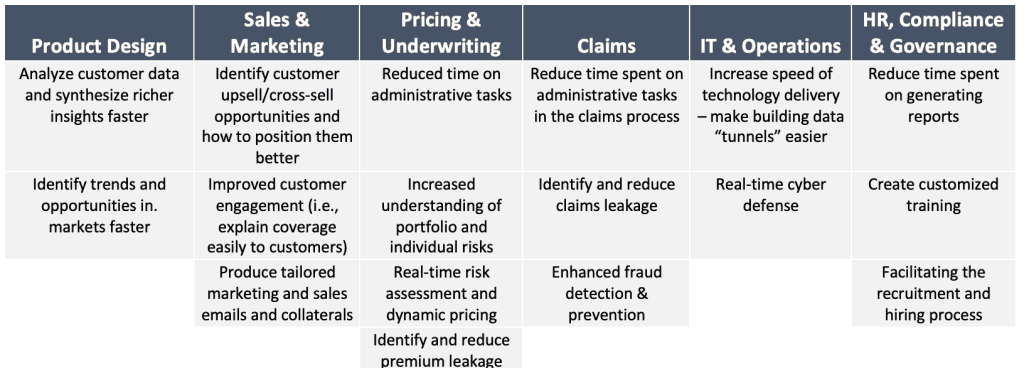
Some of these use cases are more practical today than others. Some, such as facilitating hiring and dynamic pricing, will require significant scrutiny and monitoring to manage potential biases. Some will require further advances in the core technology. However, there are also cases that can be implemented today with the right security measures. The following are some of the players in the insurance space already leveraging generative AI in some fashion:
- Cowbell, an Avanta Ventures’ portfolio company, is leveraging generative AI to deliver a real-time conversational experience for their agents and their existing policyholders.
- Zelros uses generative AI to deliver more intelligent marketing campaigns and deeper personalized recommendations at the point of sale.
- The Paladin Group in partnership with Dais Technology announced a generative AI underwriting tool earlier this year.
The darker side
The opportunities above have the potential to impact a carrier’s efficiency, profitability, and throughput. However, carriers should take note of the ways that generative AI also can be leveraged by others for malicious intent. With the democratization of AI, bad actors will also have easier access to this technology, leading to increased and improved phishing attempts and adaptive cyber-attacks. In the insurance industry, it’s important to understand how generative technology can be used to create false images and documentation for fraud. Companies are already starting to build tools to combat bad actors, such as SlashNext, which is leveraging generative AI to combat and defend against advanced business email compromise.
What is in store for the future?
While generative AI has the potential to fundamentally change how individuals and companies operate, the most immediate use cases for carriers will be those that help individuals do their jobs better and faster through human-in-the-loop automation. This includes streamlining repetitive and administrative tasks, aiding content creation, and helping users increase their throughput, such as helping underwriters process more quotes or helping sales or customer service representatives respond to more queries faster.