Artificial intelligence (AI) encompasses so many sub-segments and related markets that it’s hard to discuss AI without discussing machine learning (ML), automation, or big data. This piece is the second in a series on this broader space. The last Avanta Insights report discussed the foundation necessary to build an AI strategy – data. This article explores the broader AI/ML ecosystem, a few of the most recent trends, and the challenges that face AI implementations, especially in enterprises.
As the AI market and technology have matured, AI has permeated across every industry and into all aspects of the value chain. However, it’s also become clear that despite the prevalence of AI, there still remain significant problems and challenges to overcome for most enterprises to realize the full potential of AI. Those few enterprises that have managed to realize a greater return from AI investments and have become “AI-fueled” have kept a flexible approach to AI and have invested in the right tools, resources, a strong data foundation, and their employees. Given its limitations, for the foreseeable future, AI is a tool that needs to be intelligently applied by humans, meaning the users must understand at a basic level what is and is not possible and how to identify the right tool for their needs. If leveraged correctly, the efficiencies from leveraging AI can help companies move forward faster and have a more significant impact.
Big data, artificial intelligence, machine learning, and automation are all part of one big megatrend
In the early 2010s, big data was the big trend, followed by AI/ML. More recently, automation has become the center of attention once again. However, they are all part of one large megatrend: every company is becoming a data company, not just a software company. Data and AI are being embedded into internal processes and external applications for analytics and to improve operations. Companies are processing loans faster, responding to customers via chatbots 24/7, actively predicting churn, detecting fraud and cyber threats, and monitoring business metrics in real-time. All this innovation is powered by big data and AI/ML in concert. While each industry has had its own five minutes of fame and origin story, they have evolved in lockstep.
Despite the maturation of the ecosystem, new categories of startups continue to emerge and grow
In the past decade, the ecosystem has matured, with market leaders such as Databricks and DataRobot raising massive venture capital financings at multi-billion dollar valuations and companies such as Snowflake thriving in the public markets. Despite the presence of these AI/ML behemoths, there remain many challenges left to solve in making AI accessible, efficient, scalable, secure, and performant, as evidenced by the expanding number of AI/ML and big data-enabling companies funded by venture capitalists.
AI/ML and big data landscape (along with a sample company in each segment)
The AI market map above is just a subset of the space and ignores the nuances of specific vertical AI solutions or sub-segments of some categories listed above. Even restricting the analysis to just the categories of startups in the ML model lifecycle still produces a complex market map (see next image).
ML tools and platforms landscape
With a space as active as this, the hype cycle for trends is short as new categories are created and quickly populated by startups. One area experiencing high activity and appetite from VCs is the superset of startups that speed up or provide efficiencies pre-deployment, such as during the model training, feature engineering, data wrangling, and data cleansing. The trend is driven by the underlying issue that despite the appetite for AI/ML-driven efficiency and insight in enterprises, many AI/ML and data science projects continue to fail. In a survey conducted in June 2020, the International Data Corporation (IDC) found that 28% of AI/ML projects fail, and a 2019 Gartner report forecasted that given the rate of failure for data science projects, only 20% of analytic insights would deliver actual business outcomes. As discussed in the Insights report last quarter, having a reliable, cleansed, and well-governed data foundation is key to realizing a return from enterprises AI/ML projects.
Another trend driven by some of the behemoths in the space is functional consolidation. Companies such as Snowflake and Databricks want to become the horizontal one-stop solution for all things data and AI. In the ML tools market map above, Databricks, Dataiku, H2O.ai, and others offer capabilities across multiple categories in the ML value chain.
However, despite the breadth of their offerings, the complexity and breadth of customer needs, as well as the continued development of new features and capabilities, means that it’s likely that for the foreseeable future, companies will continue to architect their AI and data solutions leveraging numerous point product vendors. The thriving best-of-breed AI/ML companies are the ones targeting a very specific problem but integrating well into a larger AI/ML tech stack. Vertically, these are emerging companies targeting industry-specific problems with AI, such as Tractable and Claim Genius, which implement AI to expedite and quantify auto claims faster. Horizontally, these are AI/ML tools or Ops companies such as Hightouch, a company that is a reverse ETL (extract, transform and load) solution – meaning it’s taking data from data warehouses and helping to load it into operational systems (i.e., CRMs or marketing automation platforms). Due to the limitations of AI today, these narrow solutions will continue to play an essential role in the short and mid-term future of the insurance industry.
The future of artificial intelligence applications across the insurance value chain
From distribution to claims to underwriting, there are countless applications of AI/ML solutions to the insurance industry. The chart below illustrates just a few examples of use cases and benefits from leveraging AI across the insurance value chain:
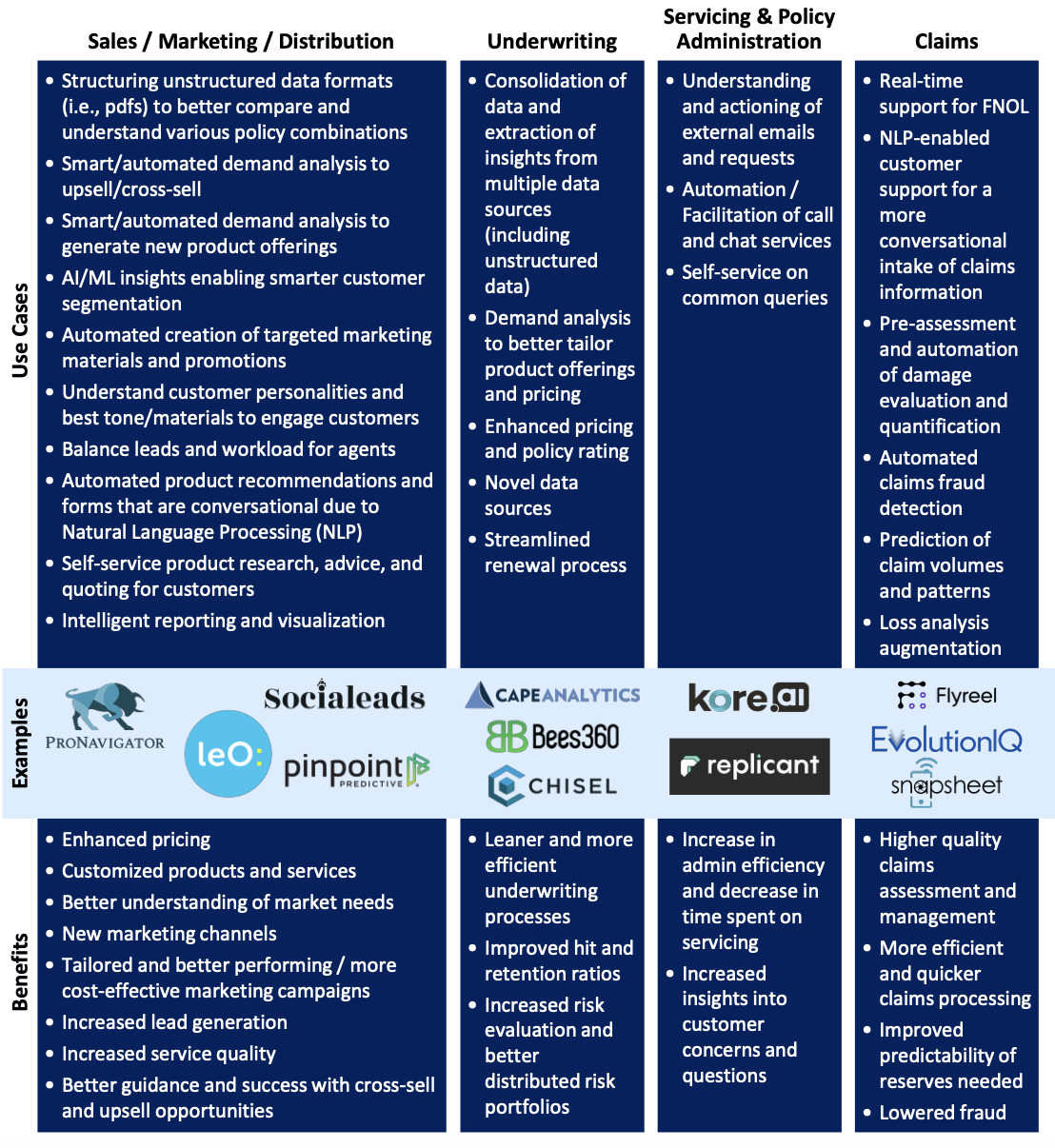
Insurance companies have progressed toward becoming more AI-enabled and literate in the past few years. However, there is still plenty of work to leverage AI throughout the enterprise and the insurance value chain. The implementation gap is not unique to the insurance industry. Some of the use cases listed in the graphic above are more prevalent and, if not already, will soon become table stakes in the insurance industry. Others, however, face numerous challenges.
Challenges facing the AI/ML ecosystem and AI implementations
As mentioned above, while companies are attempting to embed AI into internal processes, many of these projects continue to fail. Often the root causes of AI project failure are attributed to challenges in building and managing a solid data infrastructure to support the analytics and models built on top of it. There are, however, other challenges to the space:
Transparency/explainability and the trust deficit
Much of AI, particularly deep learning, is plagued by the so-called “black box” problem. The inputs and outputs are known, but the user can’t determine what happened in between to get from A (data) to B (AI model prediction). It’s particularly a challenge in regulated industries such as insurance, where organizations must show why certain decisions are being made and that they are ethical (i.e., explaining how rates are determined and what factors are used to determine insurability).
Explainable AI (XAI) is a set of tools and frameworks that can help users understand the results or outputs from an AI model and how the results were determined. It is often touted as the solution to the trust and “black box” problem. While transparency to all stakeholders and users would improve understanding of the models, it doesn’t solve everything. Knowing why the result is poor or biased doesn’t mean users will trust the AI more, nor does it mean that it can be implemented as is. Other accountability measures, such as regular auditing of the models, may need to be instituted to build trust and remove inherent biases and flaws.
Data scarcity, privacy, and security
Even if it’s not a deep learning model, a good model still requires a high volume of training data to achieve target performance. Accessing, cleansing, normalizing, and managing that data can be a significant challenge. Startups are addressing data challenges via innovative approaches. Some startups’ deep learning models are adept at mimicking training data. Other emerging companies have started to explore creating artificial or synthetic data sets; however, those are limited and are still subject to the bias of the original data set.
The data a company controls becomes ever more crucial, which leads to challenges as competitors try to gain access to new data. How can companies cooperate to share data to grow a joint partner data pool without giving away critical information? How can partners federate data, maintain security, and keep personal and identifiable information private? Data privacy and security hurdles have stymied AI/ML projects, especially in the financial, insurance, and healthcare industries.
Acceptable threshold of inaccuracy, and AI not at “human-level.”
Even today’s best AI models can fall short on accuracy and can’t always understand how to handle specific edge cases the way a human might. What’s important is determining the threshold of inaccuracy that users are comfortable with for any given problem. As a result, low-risk, low-cost use cases tend to come to fruition faster and more often, even if AI resources could have a more significant impact if applied elsewhere.
This problem relates to both the data problem and the explainability issue mentioned earlier. Organizations either need to build better and more accurate models and/or develop models that allow them to better understand how and when the models will fail.
The bias problem
One of the reasons organizations turn to machines and AI is to remove bias from decision-making. However, if the data and the decisions that the models are trained on are biased, the bias will persist in the model. Barring cultural or demographic biases, which are broader and deeper problems, data can also be biased toward preconceived notions about risk, including biases due to limited data points and context. One way to overcome bias is through data sharing – if organizations can combine and share data, they can mitigate their decision biases.
Lack of speed, computing power, and high costs
One of the limitations to getting AI/ML models up and running is the amount of computing power and time it takes to test and tweak models. For some organizations, there’s the additional challenge of how cost-prohibitive building models can be due to the sheer size and volume of data involved.
Speed and lack of compute power can sometimes cause models to take up to three months to run. Some startups have started to tackle this problem, such as SymetryML, which abstracts data sets into statistical summaries, resulting in orders of magnitude improvements in compute efficiency. However, the models that they can accelerate are limited. Some models, especially deep learning, or neural net models, which attempt to mirror the behavior of the human brain, are computationally expensive by design. They will continue to be so until computational efficiencies can be discovered or adapted to better address the models’ needs.
Fragmented tools
As mentioned earlier, most enterprises will continue to move forward with a variety of tools rather than a one-suite-solves-all approach. While the best-of-breed solution approach provides additional functionality and flexibility to solve the problems, one challenge that results from that approach is needing to find tools that integrate well with each other into a unified process.
Data and AI literacy
An enterprise’s ability to implement AI/ML projects is also highly reliant on the ability of the entire organization, not just the data scientists, to understand and work with data models. As AI/ML becomes more embedded in operations and analytics, it’s no longer just the data scientists and ML experts that need to understand the models and the outputs. Everyone in the organization that touches the data needs to become data and AI-literate. That’s where organization-wide training as well as tools and frameworks such as the AI Project Canvas can help business units work with their data scientists to better frame and identify where and how to leverage AI in the organization. It also grounds employees’ understanding of what’s possible, feasible, and viable given where AI is today.
Due to the reasons listed above and others, narrow vertical solutions with industry specialization or horizontal solutions with a constricted purview tare the solutions that continue to prosper. Both narrow, vertical AI-enabled applications and horizontal AI infrastructure solutions can still provide significant organizational value. However, the above challenges show that despite having mature players and having been “hyped” for over a decade, the AI ecosystem will continue to be an area of high activity and interest for enterprises as well as venture capitalists.
How will AI/ML impact insurance companies?
While not embedded in all internal processes today in the insurance value chain, AI will continue to permeate into processes as the technology and models mature. The rapid growth and innovation in the AI/ML market mean a rigid approach to implementing an AI strategy could cause a company to fall behind.
Realizing the full potential of AI means having a purposeful approach to identifying and structuring AI implementation pilots, taking the learnings, and sharing them across the entire organization. Learnings, whether from a failed project or a successful one, could help another project reach fruition faster. Projects will continue to fail for multiple reasons, as discussed above, but that shouldn’t deter us from continuing to experiment with and attempt to better understand AI as the field continues to progress. By becoming an AI-fueled organization, insurance companies can provide their employees with the tools and resources to serve their customers better and more efficiently.